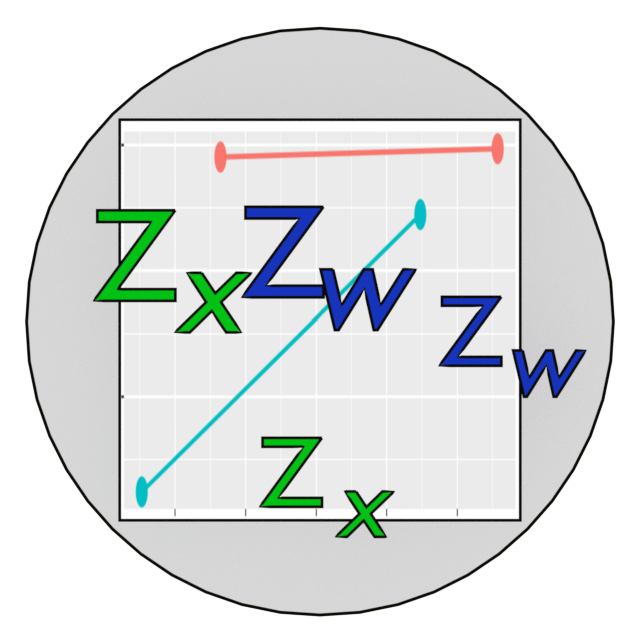
Confidence Intervals for a 'std_selected' Class Object
Source:R/confint_stdmod.R
confint.std_selected.Rd
Return the confidence intervals of estimates
in the output of std_selected()
or std_selected_boot()
.
Usage
# S3 method for class 'std_selected'
confint(object, parm, level = 0.95, type, ...)
Arguments
- object
The output of
std_selected()
orstd_selected_boot()
.- parm
The parameters (coefficients) for which confidence intervals should be returned. If missing, the confidence intervals of all parameters will be returned.
- level
The level of confidence. For the confidence intervals returned by
lm()
, default is .95, i.e., 95%. For the bootstrap percentile confidence intervals, default is the level used in callingstd_selected_boot()
. If a level different from that in the original call is specified,full_output
needs to be set in the call tostd_selected_boot()
such that the original bootstrapping output is stored.- type
The type of the confidence intervals. If est to
"lm"
, returns the confidence interval given by theconfint()
method oflm()
. If set to"boot"
, the bootstrap percentile confidence intervals are returned. Default is"boot"
if bootstrap estimates are stored inobject
, and"lm"
if bootstrap estimates are not stored.- ...
Arguments to be passed to
summary.lm()
.
Details
If bootstrapping is used to form the confidence interval by
std_selected_boot()
,
users can request the percentile confidence intervals of
the bootstrap estimates. This method does not do the bootstrapping itself.
Author
Shu Fai Cheung https://orcid.org/0000-0002-9871-9448
Examples
# Load a sample data set
dat <- test_x_1_w_1_v_1_cat1_n_500
# Do a moderated regression by lm
lm_raw <- lm(dv ~ iv*mod + v1 + cat1, dat)
summary(lm_raw)
#>
#> Call:
#> lm(formula = dv ~ iv * mod + v1 + cat1, data = dat)
#>
#> Residuals:
#> Min 1Q Median 3Q Max
#> -2146.0 -431.9 -25.0 411.2 2309.3
#>
#> Coefficients:
#> Estimate Std. Error t value Pr(>|t|)
#> (Intercept) 308.767 4075.066 0.076 0.9396
#> iv 52.760 271.242 0.195 0.8459
#> mod 5.127 40.772 0.126 0.9000
#> v1 -12.760 10.174 -1.254 0.2104
#> cat1gp2 -158.673 71.834 -2.209 0.0276 *
#> cat1gp3 -43.166 75.283 -0.573 0.5666
#> iv:mod 3.416 2.709 1.261 0.2080
#> ---
#> Signif. codes: 0 ‘***’ 0.001 ‘**’ 0.01 ‘*’ 0.05 ‘.’ 0.1 ‘ ’ 1
#>
#> Residual standard error: 665 on 493 degrees of freedom
#> Multiple R-squared: 0.6352, Adjusted R-squared: 0.6307
#> F-statistic: 143 on 6 and 493 DF, p-value: < 2.2e-16
#>
# Standardize all variables except for categorical variables.
# Interaction terms are formed after standardization.
lm_std <- std_selected(lm_raw, to_center = ~ .,
to_scale = ~ .)
# Alternative: use to_standardize as a shortcut
# lm_std <- std_selected(lm_raw, to_standardize = ~ .)
summary(lm_std)
#>
#> Call to std_selected():
#> std_selected(lm_out = lm_raw, to_scale = ~., to_center = ~.)
#>
#> Selected variable(s) are centered by mean and/or scaled by SD
#> - Variable(s) centered: dv iv mod v1 cat1
#> - Variable(s) scaled: dv iv mod v1 cat1
#>
#> centered_by scaled_by Note
#> dv 6565.02965 1094.244465 Standardized (mean = 0, SD = 1)
#> iv 15.01576 2.039154 Standardized (mean = 0, SD = 1)
#> mod 100.39502 5.040823 Standardized (mean = 0, SD = 1)
#> v1 10.13884 2.938932 Standardized (mean = 0, SD = 1)
#> cat1 NA NA Nonnumeric
#>
#> Note:
#> - Categorical variables will not be centered or scaled even if
#> requested.
#>
#> Call:
#> lm(formula = dv ~ iv * mod + v1 + cat1, data = dat_mod)
#>
#> Residuals:
#> Min 1Q Median 3Q Max
#> -1.96117 -0.39474 -0.02285 0.37579 2.11040
#>
#> Coefficients:
#> Estimate Std. Error t value Pr(>|t|)
#> (Intercept) 0.0646 0.0483 1.3385 0.18136
#> iv 0.7374 0.0274 26.9480 < 0.001 ***
#> mod 0.2599 0.0274 9.4962 < 0.001 ***
#> v1 -0.0343 0.0273 -1.2542 0.21037
#> cat1gp2 -0.1450 0.0656 -2.2089 0.02764 *
#> cat1gp3 -0.0394 0.0688 -0.5734 0.56664
#> iv:mod 0.0321 0.0255 1.2608 0.20799
#> ---
#> Signif. codes: 0 ‘***’ 0.001 ‘**’ 0.01 ‘*’ 0.05 ‘.’ 0.1 ‘ ’ 1
#>
#> Residual standard error: 0.6077 on 493 degrees of freedom
#>
#> R-squared : 0.6352
#> Adjusted R-squared : 0.6307
#> ANOVA test of R-squared : F(6, 493) = 143.047, p < 0.001
#>
#> = Test the highest order term =
#> The highest order term : iv:mod
#> R-squared increase adding this term: 0.0012
#> F test of R-squared increase : F(1, 493) = 1.5895, p = 0.208
#>
#> Note:
#> - Estimates and their statistics are based on the data after
#> mean-centering, scaling, or standardization.
#> - One or more variables are scaled by SD or standardized. OLS standard
#> errors and confidence intervals may be biased for their coefficients.
#> Please use `std_selected_boot()`.
#>
confint(lm_std)
#> 2.5 % 97.5 %
#> (Intercept) -0.03021975 0.15938347
#> iv 0.68362100 0.79114661
#> mod 0.20612513 0.31367234
#> v1 -0.08795872 0.01941694
#> cat1gp2 -0.27398880 -0.01602419
#> cat1gp3 -0.17462329 0.09572673
#> iv:mod -0.01791790 0.08209267
# Use to_standardize as a shortcut
lm_std2 <- std_selected(lm_raw, to_standardize = ~ .)
# The results are the same
confint(lm_std)
#> 2.5 % 97.5 %
#> (Intercept) -0.03021975 0.15938347
#> iv 0.68362100 0.79114661
#> mod 0.20612513 0.31367234
#> v1 -0.08795872 0.01941694
#> cat1gp2 -0.27398880 -0.01602419
#> cat1gp3 -0.17462329 0.09572673
#> iv:mod -0.01791790 0.08209267
confint(lm_std2)
#> 2.5 % 97.5 %
#> (Intercept) -0.03021975 0.15938347
#> iv 0.68362100 0.79114661
#> mod 0.20612513 0.31367234
#> v1 -0.08795872 0.01941694
#> cat1gp2 -0.27398880 -0.01602419
#> cat1gp3 -0.17462329 0.09572673
#> iv:mod -0.01791790 0.08209267
all.equal(confint(lm_std), confint(lm_std2))
#> [1] TRUE
# With bootstrapping
# nboot = 100 just for illustration. nboot >= 2000 should be used in read
# research.
set.seed(89572)
lm_std_boot <- std_selected_boot(lm_raw, to_scale = ~ .,
to_center = ~ .,
nboot = 100)
summary(lm_std_boot)
#>
#> Call to std_selected_boot():
#> std_selected_boot(lm_out = lm_raw, to_scale = ~., to_center = ~.,
#> nboot = 100)
#>
#> Selected variable(s) are centered by mean and/or scaled by SD
#> - Variable(s) centered: dv iv mod v1 cat1
#> - Variable(s) scaled: dv iv mod v1 cat1
#>
#> centered_by scaled_by Note
#> dv 6565.02965 1094.244465 Standardized (mean = 0, SD = 1)
#> iv 15.01576 2.039154 Standardized (mean = 0, SD = 1)
#> mod 100.39502 5.040823 Standardized (mean = 0, SD = 1)
#> v1 10.13884 2.938932 Standardized (mean = 0, SD = 1)
#> cat1 NA NA Nonnumeric
#>
#> Note:
#> - Categorical variables will not be centered or scaled even if
#> requested.
#> - Nonparametric bootstrapping 95% confidence intervals computed.
#> - The number of bootstrap samples is 100.
#>
#> Call:
#> lm(formula = dv ~ iv * mod + v1 + cat1, data = dat_mod)
#>
#> Residuals:
#> Min 1Q Median 3Q Max
#> -1.96117 -0.39474 -0.02285 0.37579 2.11040
#>
#> Coefficients:
#> Estimate CI Lower CI Upper Std. Error t value Pr(>|t|)
#> (Intercept) 0.0646 -0.0194 0.1257 0.0483 1.3385 0.18136
#> iv 0.7374 0.6891 0.7934 0.0274 26.9480 < 0.001 ***
#> mod 0.2599 0.1974 0.3192 0.0274 9.4962 < 0.001 ***
#> v1 -0.0343 -0.0961 0.0171 0.0273 -1.2542 0.21037
#> cat1gp2 -0.1450 -0.2710 -0.0123 0.0656 -2.2089 0.02764 *
#> cat1gp3 -0.0394 -0.1477 0.0991 0.0688 -0.5734 0.56664
#> iv:mod 0.0321 -0.0049 0.0863 0.0255 1.2608 0.20799
#> ---
#> Signif. codes: 0 ‘***’ 0.001 ‘**’ 0.01 ‘*’ 0.05 ‘.’ 0.1 ‘ ’ 1
#>
#> Residual standard error: 0.6077 on 493 degrees of freedom
#>
#> R-squared : 0.6352
#> Adjusted R-squared : 0.6307
#> ANOVA test of R-squared : F(6, 493) = 143.047, p < 0.001
#>
#> = Test the highest order term =
#> The highest order term : iv:mod
#> R-squared increase adding this term: 0.0012
#> F test of R-squared increase : F(1, 493) = 1.5895, p = 0.208
#>
#> Note:
#> - Estimates and their statistics are based on the data after
#> mean-centering, scaling, or standardization.
#> - [CI Lower, CI Upper] are bootstrap percentile confidence intervals.
#> - Std. Error are not bootstrap SEs.
#>
# Bootstrap percentile intervals, default when bootstrap was conduced
confint(lm_std_boot)
#> 2.5 % 97.5 %
#> (Intercept) -0.019442340 0.12570734
#> iv 0.689068561 0.79335143
#> mod 0.197400624 0.31922399
#> v1 -0.096125083 0.01714287
#> cat1gp2 -0.270961023 -0.01233464
#> cat1gp3 -0.147733373 0.09914400
#> iv:mod -0.004904705 0.08631912
# Force OLS confidence intervals
confint(lm_std_boot, type = "lm")
#> 2.5 % 97.5 %
#> (Intercept) -0.03021975 0.15938347
#> iv 0.68362100 0.79114661
#> mod 0.20612513 0.31367234
#> v1 -0.08795872 0.01941694
#> cat1gp2 -0.27398880 -0.01602419
#> cat1gp3 -0.17462329 0.09572673
#> iv:mod -0.01791790 0.08209267
# Use to_standardize as a shortcut
set.seed(89572)
lm_std_boot2 <- std_selected_boot(lm_raw, to_standardize = ~ .,
nboot = 100)
# The results are the same
confint(lm_std_boot)
#> 2.5 % 97.5 %
#> (Intercept) -0.019442340 0.12570734
#> iv 0.689068561 0.79335143
#> mod 0.197400624 0.31922399
#> v1 -0.096125083 0.01714287
#> cat1gp2 -0.270961023 -0.01233464
#> cat1gp3 -0.147733373 0.09914400
#> iv:mod -0.004904705 0.08631912
confint(lm_std_boot2)
#> 2.5 % 97.5 %
#> (Intercept) -0.019442340 0.12570734
#> iv 0.689068561 0.79335143
#> mod 0.197400624 0.31922399
#> v1 -0.096125083 0.01714287
#> cat1gp2 -0.270961023 -0.01233464
#> cat1gp3 -0.147733373 0.09914400
#> iv:mod -0.004904705 0.08631912
all.equal(confint(lm_std_boot), confint(lm_std_boot2))
#> [1] TRUE