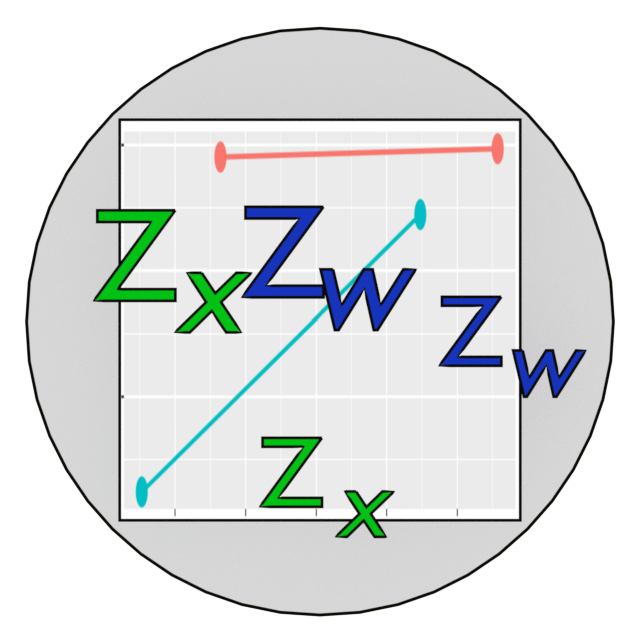
Confidence Intervals for a 'cond_effect' Class Object
Source:R/confint_condeff.R
confint.cond_effect.Rd
Return the confidence intervals of estimates
conditional effect in the output of
cond_effect()
or cond_effect_boot()
.
Usage
# S3 method for class 'cond_effect'
confint(object, parm, level = 0.95, type, ...)
Arguments
- object
The output of
cond_effect()
orcond_effect_boot()
.- parm
Ignored by this function. The confidence intervals for all available levels will be returned.
- level
The level of confidence. For the confidence intervals returned by
lm()
, default is .95, i.e., 95%. For the bootstrap percentile confidence intervals, default is the level used in callingcond_effect_boot()
.- type
The type of the confidence intervals. If est to
"lm"
, returns the confidence interval given by theconfint()
method oflm()
. If set to"boot"
, the bootstrap percentile confidence intervals are returned. Default is"boot"
if bootstrap estimates are stored inobject
, and"lm"
if bootstrap estimates are not stored.- ...
Additional arguments. Ignored.
Details
If bootstrapping is used to form the confidence interval by
cond_effect_boot()
,
users can request the percentile confidence intervals of
the bootstrap estimates. This method does not do the bootstrapping itself.
Author
Shu Fai Cheung https://orcid.org/0000-0002-9871-9448
Examples
# Load a sample data set
dat <- test_x_1_w_1_v_1_cat1_n_500
# Do a moderated regression by lm
lm_raw <- lm(dv ~ iv*mod + v1 + cat1, dat)
summary(lm_raw)
#>
#> Call:
#> lm(formula = dv ~ iv * mod + v1 + cat1, data = dat)
#>
#> Residuals:
#> Min 1Q Median 3Q Max
#> -2146.0 -431.9 -25.0 411.2 2309.3
#>
#> Coefficients:
#> Estimate Std. Error t value Pr(>|t|)
#> (Intercept) 308.767 4075.066 0.076 0.9396
#> iv 52.760 271.242 0.195 0.8459
#> mod 5.127 40.772 0.126 0.9000
#> v1 -12.760 10.174 -1.254 0.2104
#> cat1gp2 -158.673 71.834 -2.209 0.0276 *
#> cat1gp3 -43.166 75.283 -0.573 0.5666
#> iv:mod 3.416 2.709 1.261 0.2080
#> ---
#> Signif. codes: 0 ‘***’ 0.001 ‘**’ 0.01 ‘*’ 0.05 ‘.’ 0.1 ‘ ’ 1
#>
#> Residual standard error: 665 on 493 degrees of freedom
#> Multiple R-squared: 0.6352, Adjusted R-squared: 0.6307
#> F-statistic: 143 on 6 and 493 DF, p-value: < 2.2e-16
#>
out <- cond_effect(lm_raw, x = iv, w = mod)
print(out, t_ci = TRUE)
#> The effects of iv on dv, conditional on mod:
#>
#> Level mod iv Effect S.E. t p Sig CI.Lo(t) CI.Hi(t)
#> High 105.436 412.911 20.827 19.826 0.000 *** 371.991 453.831
#> Medium 100.395 395.693 14.684 26.948 0.000 *** 366.843 424.543
#> Low 95.354 378.474 19.249 19.662 0.000 *** 340.654 416.293
#>
#> [CI.Lo(t), CI.Hi(t)] shows the 95% confidence interval(s) based on t
#> statistics.
#>
#>
#> The regression model:
#>
#> dv ~ iv * mod + v1 + cat1
#>
#> Interpreting the levels of mod:
#>
#> Level mod % Below From Mean (in SD)
#> High 105.436 84.00 1.00
#> Medium 100.395 47.40 0.00
#> Low 95.354 17.20 -1.00
#>
#> - % Below: The percent of cases equal to or less than a level.
#> - From Mean (in SD): Distance of a level from the mean, in standard
#> deviation (+ve above, -ve below).
confint(out)
#> 2.5 % 97.5 %
#> High 371.9915 453.8309
#> Medium 366.8425 424.5426
#> Low 340.6543 416.2935
lm_std <- std_selected(lm_raw, to_center = ~ iv + mod, to_scale = ~ iv + mod)
# Alternative: use to_standardize as a shortcut
# lm_std <- std_selected(lm_raw, to_standardize = ~ iv + mod)
out <- cond_effect(lm_std, x = iv, w = mod)
print(out, t_ci = TRUE)
#> The effects of iv on dv, conditional on mod:
#>
#> Level mod iv Effect S.E. t p Sig CI.Lo(t) CI.Hi(t)
#> High 1.000 841.990 42.468 19.826 0.000 *** 758.548 925.431
#> Medium 0.000 806.878 29.942 26.948 0.000 *** 748.048 865.708
#> Low -1.000 771.767 39.251 19.662 0.000 *** 694.647 848.887
#>
#> [CI.Lo(t), CI.Hi(t)] shows the 95% confidence interval(s) based on t
#> statistics.
#> They should not be used when one or more variables are standardized.
#>
#> The regression model:
#>
#> dv ~ iv * mod + v1 + cat1
#>
#> Interpreting the levels of mod:
#>
#> Level mod % Below From Mean (in SD)
#> High 1.000 84.00 1.00
#> Medium 0.000 47.40 0.00
#> Low -1.000 17.20 -1.00
#>
#> - % Below: The percent of cases equal to or less than a level.
#> - From Mean (in SD): Distance of a level from the mean, in standard
#> deviation (+ve above, -ve below).
#>
#> Note:
#>
#> - The variable(s) iv, mod is/are standardized.
#> - One or more variables are scaled by SD or standardized. OLS standard
#> errors and confidence intervals may be biased for their coefficients.
#> Please use `cond_effect_boot()`.
confint(out)
#> 2.5 % 97.5 %
#> High 758.5480 925.4311
#> Medium 748.0485 865.7078
#> Low 694.6468 848.8866
# Categorical moderator
lm_cat <- lm(dv ~ iv*cat1 + v1, dat)
summary(lm_cat)
#>
#> Call:
#> lm(formula = dv ~ iv * cat1 + v1, data = dat)
#>
#> Residuals:
#> Min 1Q Median 3Q Max
#> -2457.67 -506.03 3.46 437.95 2738.18
#>
#> Coefficients:
#> Estimate Std. Error t value Pr(>|t|)
#> (Intercept) 979.20 459.19 2.132 0.0335 *
#> iv 391.03 29.25 13.370 <2e-16 ***
#> cat1gp2 -845.49 584.85 -1.446 0.1489
#> cat1gp3 259.55 620.76 0.418 0.6760
#> v1 -19.36 11.00 -1.759 0.0791 .
#> iv:cat1gp2 43.28 38.44 1.126 0.2608
#> iv:cat1gp3 -21.22 41.08 -0.516 0.6058
#> ---
#> Signif. codes: 0 ‘***’ 0.001 ‘**’ 0.01 ‘*’ 0.05 ‘.’ 0.1 ‘ ’ 1
#>
#> Residual standard error: 721.3 on 493 degrees of freedom
#> Multiple R-squared: 0.5707, Adjusted R-squared: 0.5655
#> F-statistic: 109.2 on 6 and 493 DF, p-value: < 2.2e-16
#>
out <- cond_effect(lm_cat, x = iv, w = cat1)
print(out, t_ci = TRUE)
#> The effects of iv on dv, conditional on cat1:
#>
#> Level cat1 iv Effect S.E. t p Sig CI.Lo(t) CI.Hi(t)
#> gp1 gp1 391.026 29.246 13.370 0.000 *** 333.564 448.487
#> gp2 gp2 434.302 24.937 17.416 0.000 *** 385.305 483.299
#> gp3 gp3 369.807 28.858 12.815 0.000 *** 313.107 426.506
#>
#> [CI.Lo(t), CI.Hi(t)] shows the 95% confidence interval(s) based on t
#> statistics.
#>
#>
#> The regression model:
#>
#> dv ~ iv * cat1 + v1
confint(out)
#> 2.5 % 97.5 %
#> gp1 333.5643 448.4873
#> gp2 385.3048 483.2985
#> gp3 313.1071 426.5064